Sonar Detection
Sonar (sound navigation and ranging) uses sound waves to detect objects, similar to how a bat uses echo-location to navigate, and detect objects. It is the same principle with seismic data for non-invasive underground exploration of geologic formations to locate oil or gas reserves.
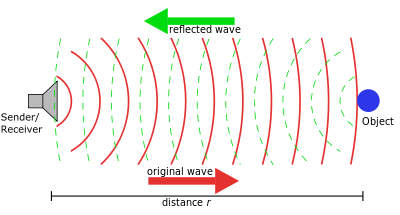
Background: A data set of sonar returns is available for rock and metal pipe with samples taken from different angles and locations. The data was collected in a laboratory under controlled conditions as a case study for detecting underground pipe. There are 111 labeled sets for the metal cylinder (pipe) and 97 sonar patterns from rocks with similar conditions. Each sample is a set of 60 numbers between 0 and 1 that represents the integrated energy within a distinct frequency band and for a given time period. | ![]() |
Although this case study is specific to detecting differences between metal pipe and rock, it is similar to detection of other underground features such as tunnels, mines, aquifers, and fluid-filled pipelines.
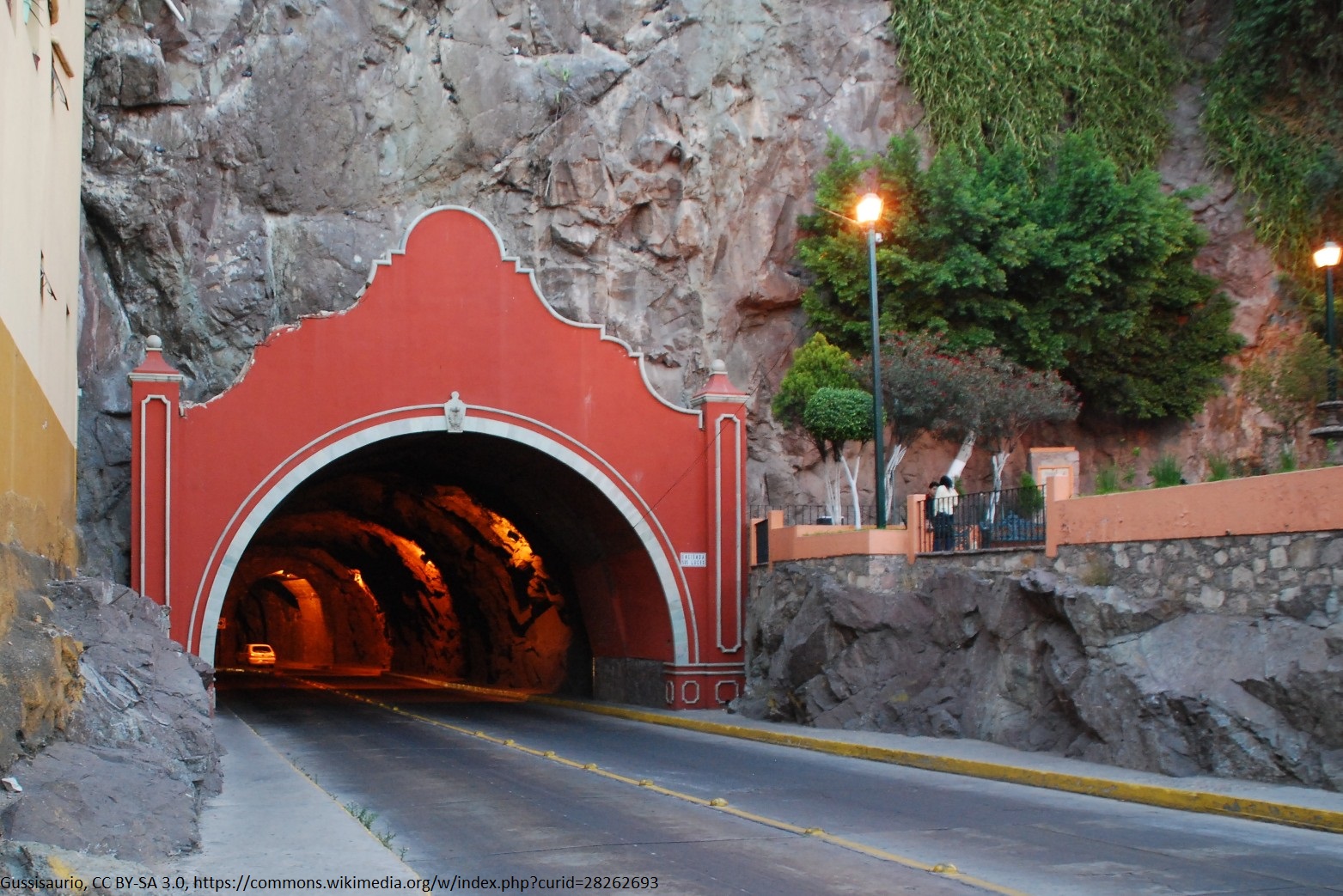
This case study focuses on classification using the 60 attributes (sonar returns) to determine whether object is rock or a metal pipe. The label associated with each record contains the letter R if the object is a rock and M if it is a metal pipe. One-hot encoding is needed to translate this character label into a binary representation (0 or 1) for classification.
url = 'http://apmonitor.com/pds/uploads/Main/sonar_detection.txt'
data = pd.read_csv(url)
# One-hot enocde 'Class' (1 is 'Metal', 0 is 'Rock') with list comprehension
data.Class = [1 if x=='M' else 0 for x in data.Class]
Objective: Develop 8 classifiers from the sonar data set. Report the confusion matrix on the test set for each classifier. Randomly select values that split the data into a train (80%) and test (20%) set by using the sklearn train_test_split with shuffle=True. Use 8 supervised learning methods of your choice. Discuss the performance of each. Submit source code and an executive summary memo (max 2 pages) of your results.
Evaluation: A confusion matrix shows true positive, false positive, true negative, and false negative groups from the test set. Generate a confusion matrix for each classifier.
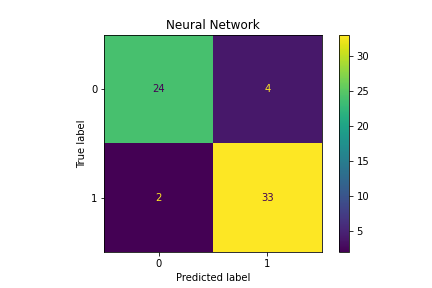
Classification: Use 8 classification methods. Possible classification methods are:
- Adaptive Boosting (AdaBoost)
- Logistic Regression
- Naïve Bayes
- Stochastic Gradient Descent
- K-Nearest Neighbors
- Decision Tree
- Random Forest
- Support Vector Classifier
- Deep Learning Neural Network
The data set may have outliers so data visualization and exploration are needed to first cleanse the data set.
Use the TCLab Data Science modules 2-5 and 7-8 (Import, Analyze, Visualize, Prepare Data, Features, Classification) as a template for analyzing and performing the classification.
References
- Gorman, R. P., and Sejnowski, T. J. (1988). Analysis of Hidden Units in a Layered Network Trained to Classify Sonar Targets, Neural Networks, Vol. 1, pp. 75-89. Article
- Connectionist Bench (Sonar, Mines vs. Rocks) Data Set, Machine Learning Repository, Center for Machine Learning and Intelligent Systems. Archive
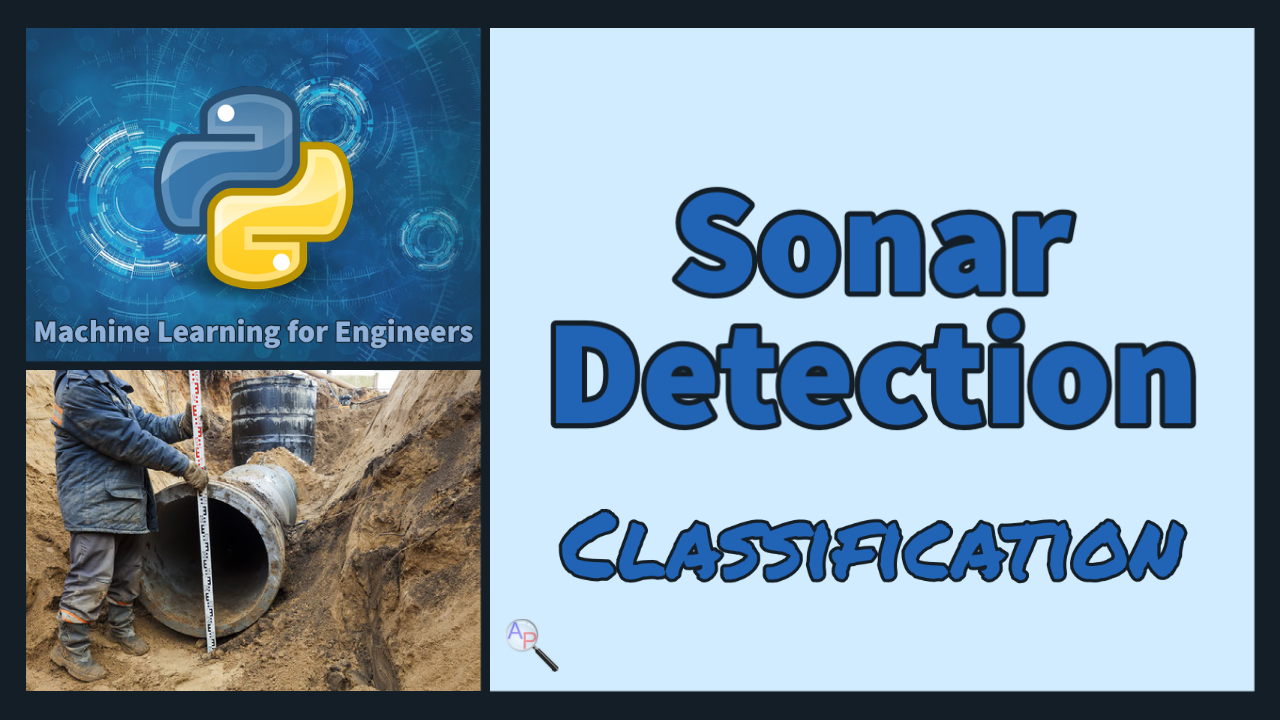
Solutions