Temperature Control Lab
The temperature control lab is an application of feedback control with an Arduino, an LED, two heaters, and two temperature sensors. The heater power output is adjusted to maintain a desired temperature setpoint. Thermal energy from the heater is transferred by conduction, convection, and radiation to the temperature sensor. Heat is also transferred away from the device to the surroundings.
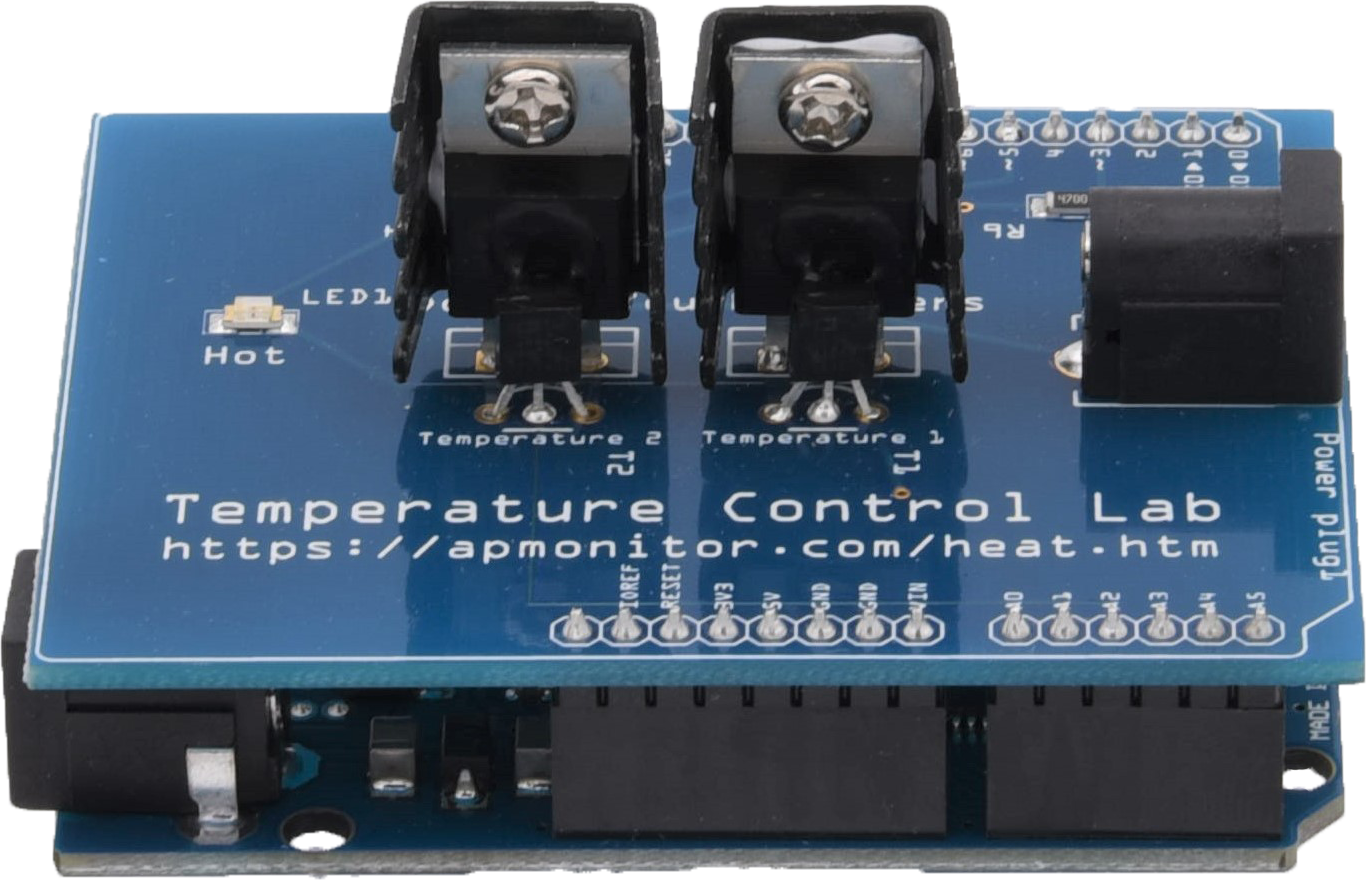
This lab is a resource for model identification and controller development. It is a pocket-sized lab with software in Python, MATLAB, and Simulink for the purpose of reinforcing control theory for students. Learning modules include:
- Begin Python with TCLab
- Learn Scientific Python for Engineers
- Process Dynamics and Control with TCLab (this course)
- Machine Learning and Dynamic Optimization with TCLab
Portable Temperature Control Lab for Learning Process Control
This lab teaches principles of system dynamics and control. In particular, this lab reinforces:
- Dynamic modeling with balance equations
- The difference between manual and automatic control
- Step tests to generate dynamic data
- Fitting dynamic data to a First Order Plus Dead Time (FOPDT) model
- Obtaining parameters for PID control from standard tuning rules
- Tuning the PID controller to improve performance

Student Resources (Web Interface, MATLAB/Simulink, and Python)
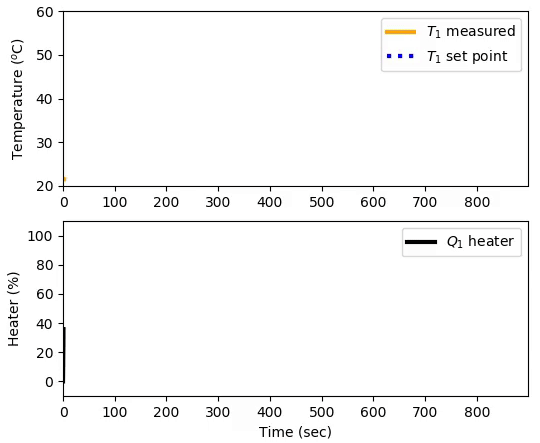
Instructor and Development Resources
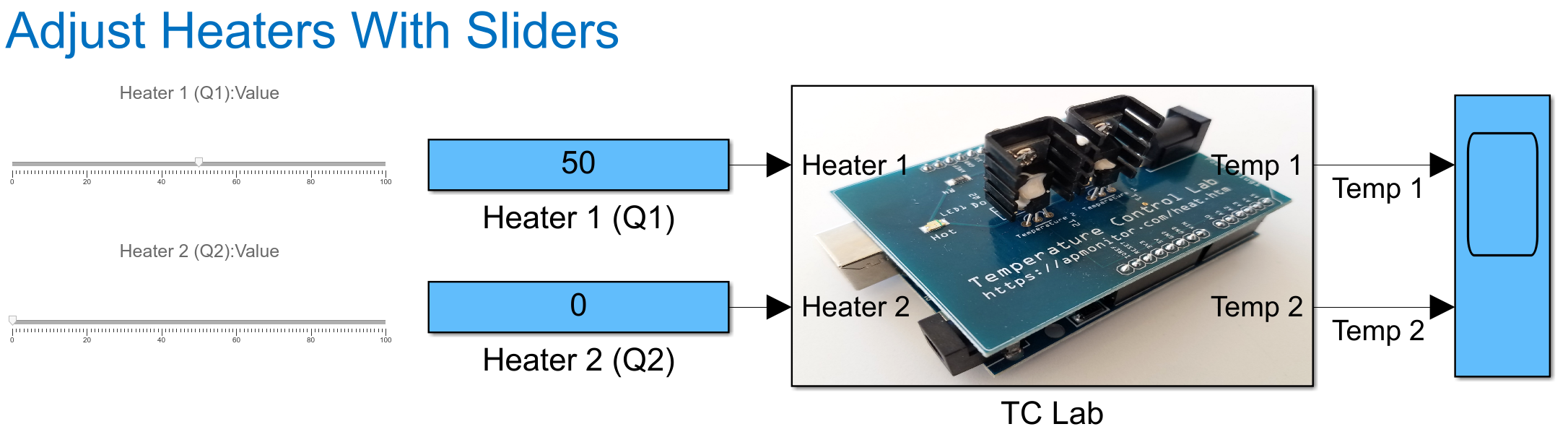
Lab Instructions
Steps 1-3 with the single or dual heater should be completed with this lab. Advanced modeling and control modules are included as additional optional information.

The TCLab Project exercises principles of modeling, estimation, and control and requires effective communication skills to convey results and deliver insights.
Problem statement: Course TCLab activities use one heater. Extend the concepts of modeling, regression, and control to derive a physics-based model of a two-heater system. Show how this first principles model compares to an empirical model. Use engineering insight to discuss fundamental factors that affect performance. Some questions to discuss may include:
- Is radiative heat transfer significant?
- Is the temperature response first order or second order?
- What disturbances affect the system?
- What physics-based parameters are uncertain and can be adjusted to match data?
- What controller tuning parameters are best?
- What controller performance criteria are used to judge best performance?
SimTune for TCLab
SimTune from APCO, Inc. provides a convenient interface to the TCLab that does not require programming in MATLAB or Python. It is a full-featured software package to implement modeling and PID tuning principles. The SimTune software has a library of simulated process scenarios for modeling and PID controller tuning. The software includes common forms learned in this course as well as common industrial forms such as Honeywell Equations (A, B, C Equations), Allen Bradley (Dependent and Independent Equations), and Control Microsystems (ScadaPack). The licensed software is freely available for those who are registered for the course, thanks to a donation by APCO, Inc.
Advanced Control Methods
The temperature control lab is also used for Advanced Estimation and Control in the Dynamic Optimization Course. The difference between the PID lab and the advanced control methods is that the model is directly used to control the process versus only for tuning correlations. This approach is called Model Predictive Control (MPC) because the simulated system is driven to a desired set point with the use of an optimizer. Also, instead of estimating the model once from step tests, Moving Horizon Estimation (MHE) updates the model with every new measurement. The updated model is transferred to MPC for improved performance through adaptive control.
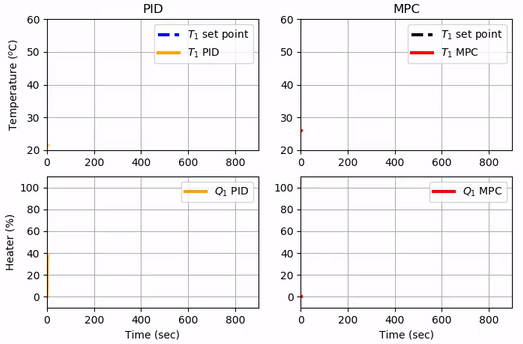
References
- Oliveira, P.M. Cardoso, A., Soares, F., Machado, J., SΓ‘, J., Lopes, H., Silva V., Temperature Control Laboratory (TCLab): Demonstration of Use in Portugal, 2023 6th Experiment@ at International Conference (exp.at'23), Γvora, Portugal, 2023, pp. 13-14, DOI: 10.1109/exp.at2358782.2023.10546110. Article
- Patel, K.M., A practical Reinforcement Learning implementation approach for continuous process control. Computers & Chemical Engineering, 2023, 174, p.108232. Article
- Lee, S., Kang, J.W. Deep Learning Model Comparison Study on Temperature Control in Electric Facilities. J. Electr. Eng. Technol, 2022. Article
- Moura Oliveira, P.B., Soares F., Cardoso A., Pocket-Sized Portable Labs: Control Engineering Practice Made Easy, IFAC Symposium on Advances in Control Education 2022, Hamburg, Germany, 24-27 July 2022. IFAC-PapersOnLine, Vol. 55, Issue 17, pp. 150-155. Article
- MejΓa, C., Salazar, E., Camacho, O., A comparative experimental evaluation of various Smith predictor approaches for a thermal process with large dead time, Alexandria Engineering Journal, Volume 61, Issue 12, 2022, Pages 9377-9394, ISSN 1110-0168, DOI: 10.1016/j.aej.2022.03.047, 2022. Article
- Yerolla, R., Besta, C.S., Development of tuning free SISO PID controllers for First Order Plus Time Delay (FOPTD) and First Order Lag Plus Integral Plus Time Delay model (FOLIPD) systems based on partial model matching and experimental verification, Results in Control and Optimization, 2021, ISSN 2666-7207, 2021. Article
- Sharma, S., Padhy, P.K., An indirect approach for online identification of continuous time-delay systems, Int. Journal of Numerical Modelling, 30 Aug 2021, doi: 10.1002/jnm.2947 Article
- Hedengren, J.D., Teaching Dynamics and Control with Arduino-based TCLab, Invited Presentation at MathWorks Special Session, 59th Conference on Decision and Control, Jeju Island, Republic of Korea, December 14-18, 2020. Presentation
- Moura Oliveira, P.B., Hedengren, J.D., Solteiro Pires, E.J., Swarm-Based design of Proportional Integral and Derivative Controllers using a Compromise Cost Function: An Arduino Temperature Laboratory Case Study, Special Issue: Algorithms for PID Controller, Algorithms, 13(12), 315, DOI: 10.3390/a13120315, 2020. Article
- Hedengren, J.D., Kantor, J., Computer Programming and Process Control Take-Home Lab, Computer Aids for Chemical Engineering (CACHE) News, Summer 2020. Article
- Moura Oliveira, P., Hedengren, J., Rossiter, J.A., Introducing Digital Controllers to Undergraduate Students Using the TCLab Arduino Kit, 21st IFAC World Congress, Berlin, Germany, July 12-17, 2020. Article
- Moura Oliveira, P., Hedengren, J., Boaventura, J., Bridging Theory to Practice: Feedforward and Cascade Control with TCLab Arduino Kit, 14th International Conference on Automatic Control and Soft Computing (CONTROLO), BraganΓ§a, Portugal, July 2020. Proceedings
- Park, J., Martin, R.A., Kelly, J.D., Hedengren, J.D., Benchmark Temperature Microcontroller for Process Dynamics and Control, Computers & Chemical Engineering, Special Issue in Honor of Thomas F. Edgar, 135, 6 April 2020. Preprint | Article
- Rossiter, J.A., Jones, B.L., Pope, S., Hedengren, J.D., Evaluation and Demonstration of Take Home Laboratory Kit, Invited Session: Demonstration and poster session, 12th IFAC Symposium on Advances in Control Education, July 7-9, 2019, 52 (9), pp. 56-61, Philadelphia, PA, USA. Preprint
- Hedengren, J.D., Martin, R.A., Kantor, J.C., Reuel, N., Temperature Control Lab for Dynamics and Control, AIChE Annual Meeting, Orlando, FL, Nov 2019. Abstract
- Moura Oliveira, P., Hedengren, J.D., An APMonitor Temperature Lab PID Control Experiment for Undergraduate Students, 24th IEEE Conference on Emerging Technologies and Factory Automation (ETFA), Sep 10th - 13th, 2019, pp. 790-797, Zaragoza, Spain. Preprint | Paper
- Park, J., Patterson, C., Kelly, J., Hedengren, J.D., Closed-Loop PID Re-Tuning in a Digital Twin By Re-Playing Past Setpoint and Load Disturbance Data, AIChE Spring Meeting, New Orleans, LA, April 2019. Abstract
Additional Activities
Additional activities are available with each homework assignment in the Process Dynamics and Control Course. The TCLab assignments are listed in the right column of the course schedule with the associated background lecture material and the simulation assignment in the other columns. The TCLab assignments reinforce lecture material with TCLab activities.
Model Development
- Simulate Step Response
- Convective Heat Transfer
- Radiative Heat Transfer
- Linearize Energy Balance
- First Order Plus Dead Time (Graphical)
- First Order Plus Dead Time (Regression)
- Impulse Response (Analytic Solution)
- State Space Simulation
- Second Order Response
- Higher Order Response
Control Development
- Controller Design
- P-Only Control
- PI Control
- PID Control
- PI Control Tuning
- PID Control Tuning
- PID with Feedforward
- Heater Actuator
- Temperature Sensor
- Block Diagram
- On/Off Control
- Stability Analysis
- Cascade Control